
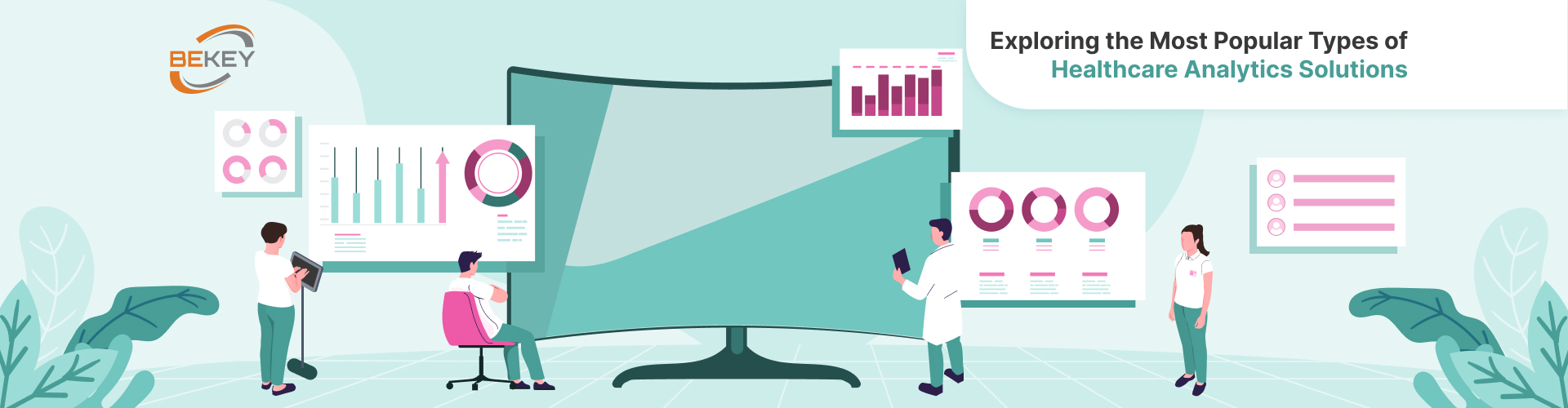
Exploring the Most Popular Types of Healthcare Analytics Solutions
- Descriptive Analytics: Unveiling the Past in Healthcare
- Predictive Analytics: Illuminating the Future of Healthcare
- Prescriptive Analytics: Shaping Optimal Healthcare Decision-Making
- Real-Time Analytics: Empowering Timely Interventions in Healthcare
- Clinical Decision Support Systems (CDSS): Empowering Evidence-Based Healthcare Decisions
- Challenges of Data Analytics in Healthcare
- Conclusion
The healthcare sector witnesses a vast influx of information on a daily basis, encompassing patient records, clinical research, and administrative data. This abundance of data originates from diverse channels, including electronic health records (EHRs), medical imaging devices, wearable gadgets like smartphones and watches, and many other digital resources.
In order to extend the value of this healthcare data beyond its initial purpose, it necessitates further analysis. Currently, the industry is experiencing a pressing demand for data analytics. Medical professionals and researchers can uncover concealed patterns and trends within clinical data by employing it. This valuable insight, in turn, facilitates enhanced patient care, improved treatment efficacy, and cost-saving opportunities, and promotes innovation.
Descriptive Analytics: Unveiling the Past in Healthcare
Descriptive analytics is a crucial aspect of healthcare, enabling organizations to extract valuable insights from historical data. It focuses on summarizing past events and trends, offering a comprehensive understanding of the healthcare ecosystem. Key focus areas include patient demographics, disease prevalence mapping, and resource utilization analysis.
Patient demographics analysis involves studying factors like age, gender, ethnicity, and socioeconomic status to gain insights into specific patient groups’ needs. Disease prevalence mapping helps identify which diseases disproportionately affect certain regions or communities by analyzing past healthcare data. Resource utilization analysis utilizes historical data on resource usage, such as hospital beds, equipment, and staff allocation, to optimize operations and enhance efficiency.
Predictive Analytics: Illuminating the Future of Healthcare
Predictive analytics for healthcare has transformed the sphere by enabling organizations to project future events and outcomes based on historical data and advanced statistical models. It utilizes diverse data sources, such as EHRs, medical imaging, and patient-generated data, to create complex models that analyze patterns and establish predictive relationships. This empowers healthcare providers to anticipate events with precision, particularly in early disease identification and risk prediction for readmission. Additionally, predictive analytics facilitates patient deterioration monitoring by analyzing vital signs, lab results, and clinical notes. Its applications extend to healthcare operations, resource allocation, and decision-making processes.
Prescriptive Analytics: Shaping Optimal Healthcare Decision-Making
Unlike descriptive and predictive analytics, which focus on understanding the past and predicting the future, prescriptive one goes a step further by providing recommendations on what actions should be taken to achieve desired outcomes.
Prescriptive analytics in healthcare has a significant influence by enhancing treatment plans. By examining patient information, such as their medical background, genetic data, treatment results, and reactions to therapies, prescriptive analytics models can produce individualized suggestions for treatment. These recommendations take into account individual patient characteristics, such as genetic predispositions, comorbidities, and lifestyle factors, to optimize treatment efficacy and minimize adverse effects.
Real-Time Analytics: Empowering Timely Interventions in Healthcare
Prescriptive analytics goes beyond understanding the past and predicting the future by providing actionable recommendations to achieve desired outcomes. In healthcare, it greatly impacts treatment plans by analyzing patient information such as medical history, genetic data, treatment results, and therapy responses. Personalized treatment suggestions are generated, considering individual patient characteristics to enhance effectiveness and minimize adverse effects. Leveraging these insights enables healthcare providers to improve treatment outcomes, optimize resource allocation, and enhance operational efficiency.
Clinical Decision Support Systems (CDSS): Empowering Evidence-Based Healthcare Decisions
Clinical Decision Support System utilizes extensive patient data, including medical records, lab outcomes, imaging findings, and research literature, to provide healthcare practitioners with timely and relevant information during patient treatment. CDSS generates suggestions, notifications, and prompts aligned with clinical guidelines and standards by employing advanced algorithms and decision-support tools.
One key function of CDSS is drug interaction checking. It analyzes a patient’s medication profile and cross-references it with a comprehensive database to flag potential interactions, contraindications, and allergies. It also aids diagnosis support by analyzing symptoms, medical history, and test results. It presents clinicians with differential diagnoses, suggests additional tests or screenings, and provides relevant clinical knowledge to support decision-making.
Furthermore, CDSS assists in selecting treatment options, adjusting medication dosages, and considering potential risks. It improves treatment outcomes, promotes standardized care, and prioritizes a patient-centric approach to healthcare delivery.
Challenges of Data Analytics in Healthcare

Several significant challenges hinder the effective implementation of data analytics in healthcare organizations. Let’s explore them and their impact:
Unstructured Data
Most healthcare data is unstructured, originating from natural language processing and lacking standardization.
Fragmented and dispersed data within and across organizations make aggregating and analyzing unstructured data difficult.
The lack of interoperability in electronic health records (EHRs) further complicates data sharing and analysis.
Missing Data & Data Sparsity
Inadequate data collection or lack of documentation can lead to missing electronic medical record (EMR) data.
Data sparsity arises from limited hospital visits and incomplete recording of medical features.
Techniques such as imputation methods can handle missing data and address sparsity.
Security & Privacy Concerns
Data analytics in healthcare raises privacy concerns, especially with the Health Insurance Portability and Accountability Act (HIPAA) legislation.
Open-source platforms increase vulnerability to breaches, and centralized storage increases the risk of cyber-attacks.
Ensuring data privacy and security is crucial when implementing healthcare data analytics solutions.
Data Standardization
Lack of standardization and compatibility between EHR platforms pose a challenge for data analytics in healthcare.
Non-compatible formats and lack of standardization complicate data acquisition and cleansing.
Standards organizations like Health Level Seven International (HL7) develop global interoperability standards like FHIR to address data standardization issues.
Data Irregularity
EMR data irregularity stems from records being recorded only during hospital visits, resulting in unevenly spaced time spans.
Analyzing and extracting insights from such data is challenging due to varying granularity and time intervals between visits.
Approaches like establishing a common baseline, data transformation, and developing models for irregular data can help overcome this challenge.
Data Bias
Biased sampling and incomplete data collection can introduce biases in healthcare data analytics.
Patient health status and the doctor’s judgment may lead to imbalanced data sampling.
Patients seeking care from multiple organizations or incomplete medical examinations can contribute to biased conclusions.
Data Storage & Transfers
The cost of securing and storing generated data is high compared to data generation itself.
Transferring data between locations and analyzing it incurs additional expenses.
Cloud-based health information technology adds security concerns during data extraction, transformation, and loading.
Managing Unstructured Data
Extracting meaningful insights from unstructured data requires advanced techniques like text mining, natural language processing, and image recognition.
Due to their unstructured nature, clinical notes, research articles, and medical images pose challenges in the analysis.
By addressing these challenges, healthcare organizations can unlock the potential of data analytics, leading to improved patient outcomes and enhanced healthcare operations.
Conclusion
The utilization of healthcare analytics has emerged as a revolutionary influence in the contemporary healthcare arena, enabling organizations to use insights derived from data and make well-informed choices. By embracing these tools, medical establishments can tap into the potential of data to streamline clinical workflows, enhance patient outcomes, and improve operational efficiency. The incorporation of healthcare analytics solutions holds significant promise in reshaping healthcare delivery. Through constant exploration, innovation, and adoption of these solutions, we can unlock uncharted territories in personalized medicine, population health management, and optimization of healthcare systems. Let us embark on this endeavor of harnessing the capabilities of healthcare analytics solutions to pave the way toward a healthier future.
Faq
What are healthcare analytics solutions?
+Healthcare analytics solutions utilize various sources of information, including patient characteristics, medical records, clinical trials, and billing data, to help healthcare providers identify potential risks, improve treatment strategies, allocate resources more effectively, and implement practices based on solid evidence. The ultimate goal of these solutions is to improve the quality of care and achieve better healthcare results for both individuals and larger groups of people.
What are the benefits of using healthcare analytics solutions?
+Healthcare analytics solutions enhance decision-making by providing data-driven insights and optimizing resource allocation. These solutions streamline operations, increase efficiency, and reduce costs. Analytics solutions enhance population health management by identifying high-risk individuals. They facilitate personalized medicine through predictive modeling and precision diagnostics.
What are the popular types of healthcare analytics solutions?
+Popular types of analytics solutions include:
Descriptive: Summarizes past data to provide insights into patient demographics and healthcare costs.
Predictive: Uses statistical algorithms to forecast future outcomes.
Prescriptive: Recommends optimal actions by combining predictive analytics with decision-making algorithms.
Clinical: Focuses on patient-specific data to improve individual care.
How are healthcare analytics solutions used in population health management?
+Healthcare analytics solutions utilize advanced algorithms to identify patterns, trends, and insights. By leveraging this information, healthcare providers can identify high-risk populations, predict disease outbreaks, and assess the effectiveness of interventions. Analytics solutions also facilitate care coordination, enabling healthcare teams to ensure seamless patient transitions.
Authors
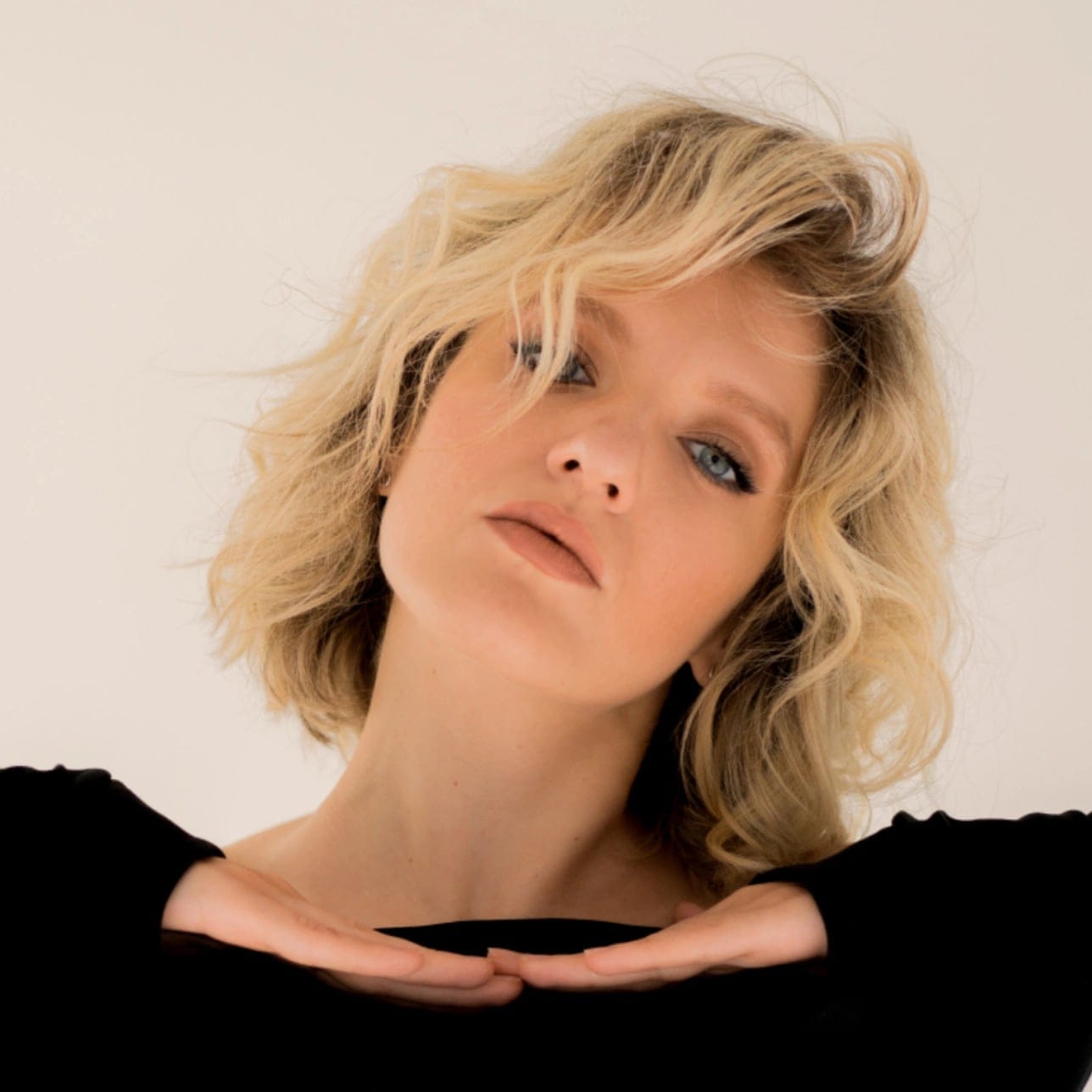
Tell us about your project
Fill out the form or contact us
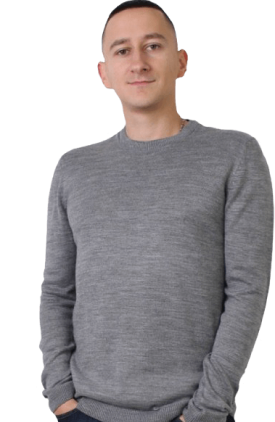
Related posts
Tell us about your project