
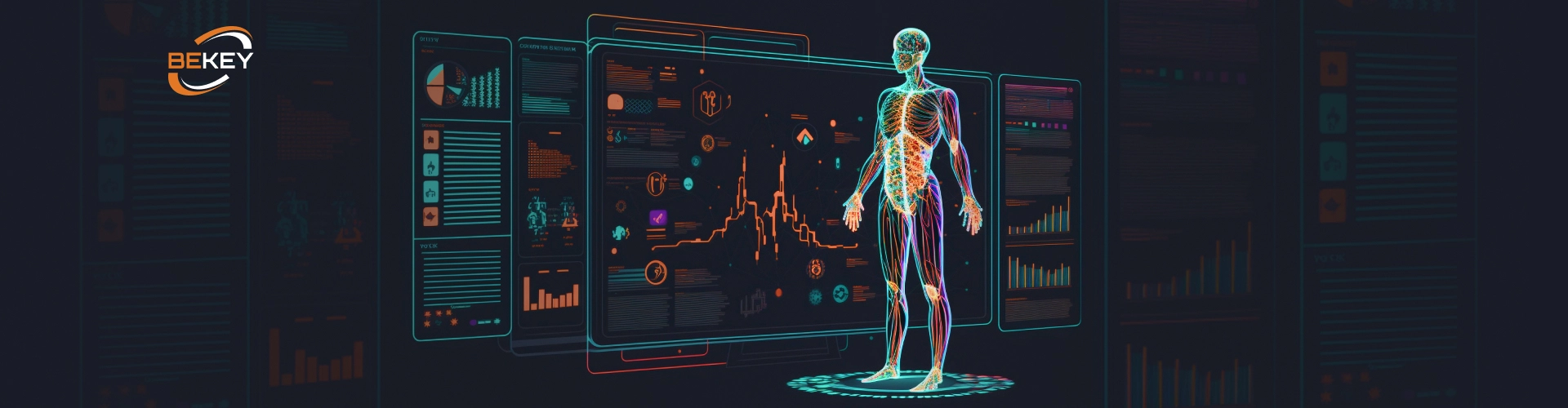
How Big Data Analytics Helps Digital Healthcare
- Why does healthcare change?
- What is Big Data Analytics?
- Big data analytics consists of
- Towards clinical and patient safety through big data analytical solutions
- Big data analytics for financial security operations in healthcare providers
- Transforming through analytics is tough. How to deal with challenges?
In 2019, as the crazy tide of hype around big data calmed down, industries are facing a challenge of properly utilizing it. Healthcare, being a bit rigid domain itself — all these regulations, laws, and an underlying silo mentality — is among them. To survive digital transformation, healthcare — now more than ever — needs to improve patient outcomes and increase the value of treatment. Data analytics gives clinicians tools to do it.
We’ll talk about analytical methods and tools healthcare providers build to help clinicians transform healthcare into a service industry: establish better care, communication, and reduce the costs of treatment. Before that, though, let’s answer two questions: first, how is the Healthcare industry doing; second, what the big data analytics is.
Why does healthcare change?
“I’m infuriated at a medical research model that has become so corrupted by the corrosive influence of big money that it’s completely lost sight of its primary objective: finding ways to eradicate diseases, not fancy new formulas for turning them into cash cows,” wrote a person with multiple sclerosis on their blog called “Wheelchair Kamikaze”. That quote alone answers questions about the primary reasons for changes in healthcare. Nonetheless, let’s take a more detailed view.
Previously, all medical institution used the fee-for-service (FFS) approach when working with patients. Patients paid for different medical services (consultation, lab tests, surgeries, etc.) separately, and the volume of treatment — the number of services, given — defined the overall cost of it. Such a way encouraged healthcare providers to do more work as they were paid on the quantity of it.
However, more isn’t always better or necessary.
Dartmouth Atlas Project outlined a paradoxical, but an unsurprising flaw of the FFS method: “Patient does not experience improved survival or better quality of life if they live in regions with more care. In fact, the care they receive appears to be worse.”
Along with Not Helping and Making Things Worse, FFS was and still is, ridiculously expensive due to the handful of irrelevant services. It became clear that the situation must change.
Luckily for everyone in the healthcare industry, digital transformation provided clinics, third-party vendors, and insurers with a hint of extra motivation. To survive in the competitive race, they had to change their approach to patient care and, well, start making things better.
So the shift to value-based care (VBC) began. What is it? It’s an approach where the financial reward for treatment is based on patient health outcomes: valuable treatment equals significant profits. Valuable treatment, though, is a personalized, individual for every patient, course of dealing with an illness — that includes right tests and right medications, which are cost-effective and which fit specifically into the given patient’s case.
To achieve that accuracy, healthcare started working with big data.
What is Big Data Analytics?
Big data is a vast scope of unstructured data, that “requires specific technology and analytical methods for its transformation into value.” In healthcare, data originates in Electronic Health Records (EHRs, or Electronic medical records) that consist of clinical notes and lab results; in billing information; in prescriptions; etc. This data extends a basis for big data analytics.
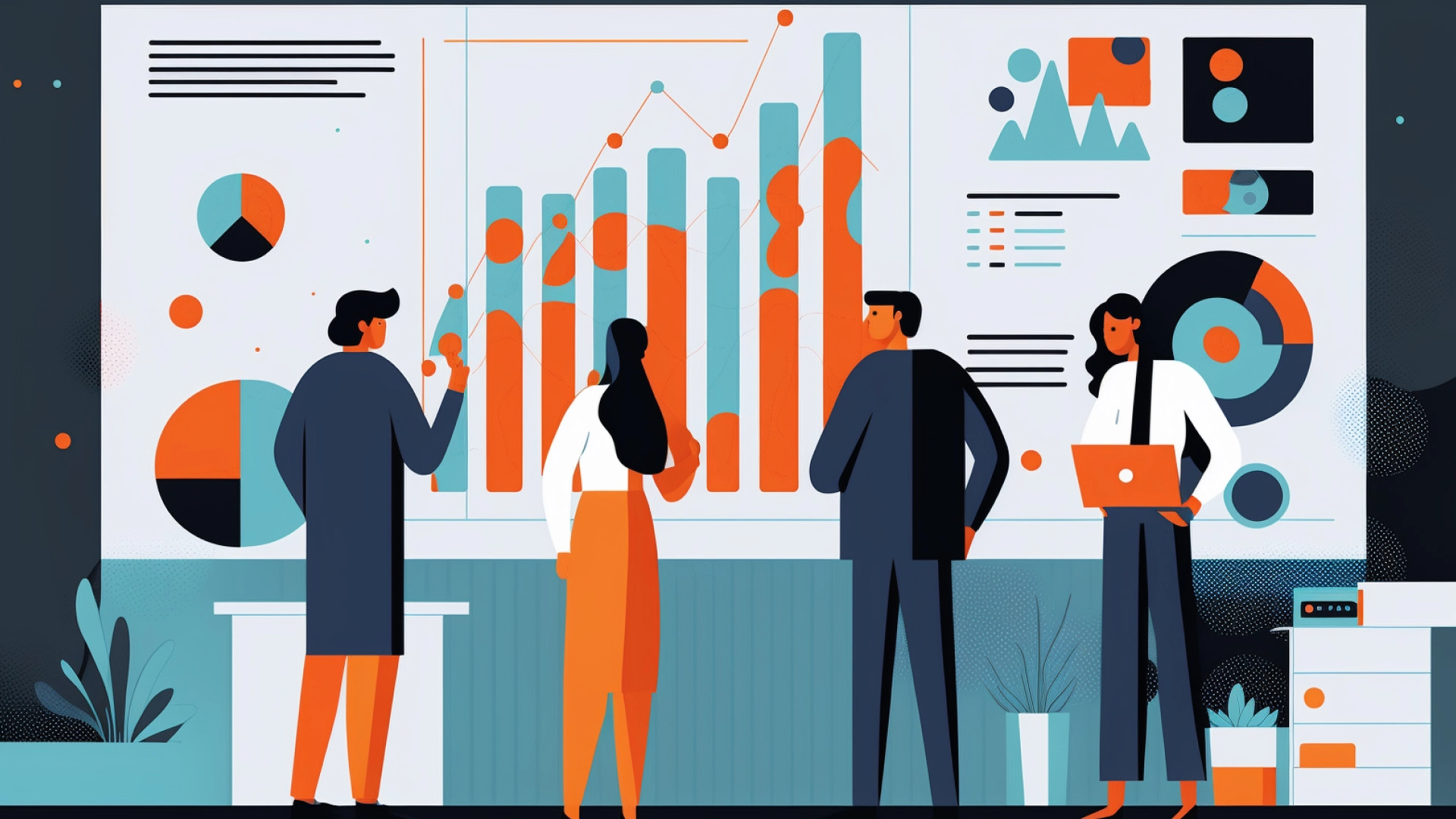
Big data analytics consists of
- descriptive (what data is)
- predictive (how data will change in the future), and
- prescriptive types of analytics (how an organization can use these predictions or patterns to improve their operations and business outcomes).
Analytical assets include machine learning algorithms. These that can look through the large scope of data, “memorize” it and its patterns that go unnoticed by humans and offer insights that forecast the future behaviour of data and outcomes of every possible decision. Results of such analysis depends on the type of algorithm — we’ll discuss them later in the article.
Machine learning is one of the methods to build artificial intelligence (AI). AI uses these algorithms to automatically process tons of data, learn from it, and help humans notice things they wouldn't notice otherwise. Thus, data-driven predictions of treatment outcomes, more accurate decision making, fewer risks and leg work.
Towards clinical and patient safety through big data analytical solutions
Precision medicine is, by definition of Precision Medicine Initiative, “an emerging approach for disease treatment and prevention that takes into account individual variability in genes, environment, and lifestyle for each person.” It’s like personalized ads, but more useful.
Precision medicine was a big deal for healthcare for a long time: sports genetics used it to adjust the genetic traits of an athlete for a fitting sport—for instance, it defined whether he or she will be more effective, in sprints of in marathons. Not all the industry is trying to implement that paradigm—it’s one of the pillars of value-based care.
How exactly is it integrated into patient care solutions in clinics?
Clinical Decision Support (CDS)
The core of precision medicine is clear, data-driven insights on how to improve treatment or prevent illnesses. Predictive algorithms make it possible; systems they’re embodied into are called clinical decision support systems (CDS). CDSs augment the power of the machines and the power of healthcare workers to make patients’ lives better.
They can provide physicians with recurring reminders about patients’ treatment, help them figure out how rare cases of common diseases can be treated, check which drug and what dosage are better for a particular patient, screen X-ray X-ray photos and MRI scans for abnormalities.
In a perfect world, CDSs process all the data connected to patients and diseases and provide physicians with immediate and accurate solutions aimed to help their patients recover. The world isn’t perfect yet. The majority of hospitals alarms is unreliable, useless, and clinicians are sick of them so much that they come up with a name for it: alarm fatigue. Not helpful, distractive, makes them hate their jobs. The situation is slowly changing though, so let’s take look at the good things.
Example. Working on the case of Valley Health System, Databank, top CDS in the USA, developed a solution called Alertspace. Integrated into the hospital’s systems, Alertspace provides clinicians with relevant alerts about patients who need their immediate attention and who could be assessed later. Updates in drug information are uploaded to the EHR automatically. Alert management is customized and actually helpful: clinicians choose what alerts they need to receive, they control their own workflows and distractions. Levels of hating a job due to alarm fatigue decreases and clinicians become happier.
Diagnosis assistance
Diagnosis assistance solutions use machine learning to help doctors draw out more precise diagnosis and the more effective treatment for a particular condition. They forecast these on the basis of hospital and patient data, or life sciences research.
Some of clinical support analytical algorithms use natural language processing (NLP). They comprehend text records of a current patient’s data with other data in EHRs where the same semantics is used. The interface can look like a dashboard or a question-answering system: in it, a doctor can ask a question and get a prioritized, science- and previous practice-based set of most likely answers with real-world evidence.
Example. Aeiton’s solution for precise critical decision making in choosing treatment and a type of medication. It’s a platform that provides healthcare with treatment efficacy analytics that’s done not on the basis of randomized clinical trials (which is an ancient, but still valid healthcare method of data analysis), but on the scope of real-world patient data from EHRs, claims, and patient feedback (!). Thus, hospitals are able to treat efficiently and safely.
Preventive and critical interventions
According to PwC research, more than half of healthcare customers would agree to use FDA-approved digital app or software that allows improving their healthcare condition. Wearable devices for remote monitoring, mobile apps and chats for clinics boost customer satisfaction and give providers an opportunity to obtain constant data flow about patient condition and illness development.
Now, wearable devices and hospital-placed remote monitoring solutions help medical providers to put their patients on 24-hours surveillance. Ok, perhaps, it sounds like something from a Bond movie, but the value of patient care that’s enabled by them is hard to overestimate.
Wearables collect patient health vital data, such as heart rate, blood pressure, or level of sugar in the blood and present them to the clinicians on a dashboard. With these data, after-surgery complications, unexpected reactions on medications, or, for instance, in-hospital infection (that are very common) can be noticed and addressed early on.
Also, such solutions help understand illnesses in individuals better: diabetes, for instance, progresses differently in different people, and if clinicians see the real-time data, it’s easier for them to deal with risks quicker.
Example. BeKey’s remote monitoring solution for ALTASense Biomed is a wearable multi-sensing device which sends vital signs of elderly and chronically ill patients to clinicians so that they can monitor their patients' safety and assess risks faster.
Care and communication management
Communication is a key, and talking to patients is extremely important for healthcare workers. Apps for clinics or chats, integrated into a system that monitors patients’ health condition, are aimed at improving it. Patient-friendly portals and apps like eHealth System, on other hand, show patients their medical records, so they understand what’s going on, what they need to do and how much are they going to pay for it. They have a calming effect and increase patient education and satisfaction. Ok, but what about data?
Communication platforms are a source of valuable socioeconomic information and patient feedback. Latter is often used for NLP sentiment analysis. Using the algorithm, the care manager is able to find similar cases and give a recommendation on a diagnosis or connect patients to a physician, specialized in treating their condition specifically, in mere seconds. Which means, less anxiety for patients.
Care management software also includes platforms that help clinical staff utilize the true art of time-management. These automatically remind nurses and doctors about patients’ meds schedule, further or recurrent procedures, admissions or readmission, etc., so their workflow is organized better.
Example. Rounding platform by cipherhealth.com allows clinicians to exchange information about patients’ needs, requests, and responsibilities. It makes interactions between patient and hospital more visible and understandable for hospitals and generates alerts on patients medication. In such a way, the platform drives productivity and satisfaction in nurse teams. Also, it has a place for patient feedback on the quality of services.
Population health management
Through analytical solutions, clinics can use the latest scientific research and population health data from other healthcare providers, life scientists, and pharma companies, to figure out patterns, anomalies, and unusual traits in historical data of their patients.
These algorithms help make better prescriptions on the basis of trends and tendencies in patients’ previous interactions with pharma. Considering the efforts of American healthcare to beat opioid addiction, such solutions are essential. More global population health solutions help hospitals predict epidemic breakouts and prepare for them in the real-time mode!
Example. Bamboohealth solution for healthcare providers, NarxCare, gives clinicians patients’ prescription history for the last two years, including information from providers, pharmacies, quantities, and active prescriptions. Integrated into hospitals’ EHR, it demonstrates specific risks aligned with types of meds (painkillers, sedatives, stimulants.). Physicians can make fully informed decision and control substance medication abuse more efficiently.
Big data analytics for financial security operations in healthcare providers
Big data analytics also improves the safety of patient care. The “how” is easy. Algorithms don’t have a short attention span, they cannot be tired or distracted — so there are fewer medical errors. Additionally, machine learning algorithms help reduce losses and mistakes in hospital claims, create patient treatment plans that are both cost-effective and personalized and check compilability automatically.
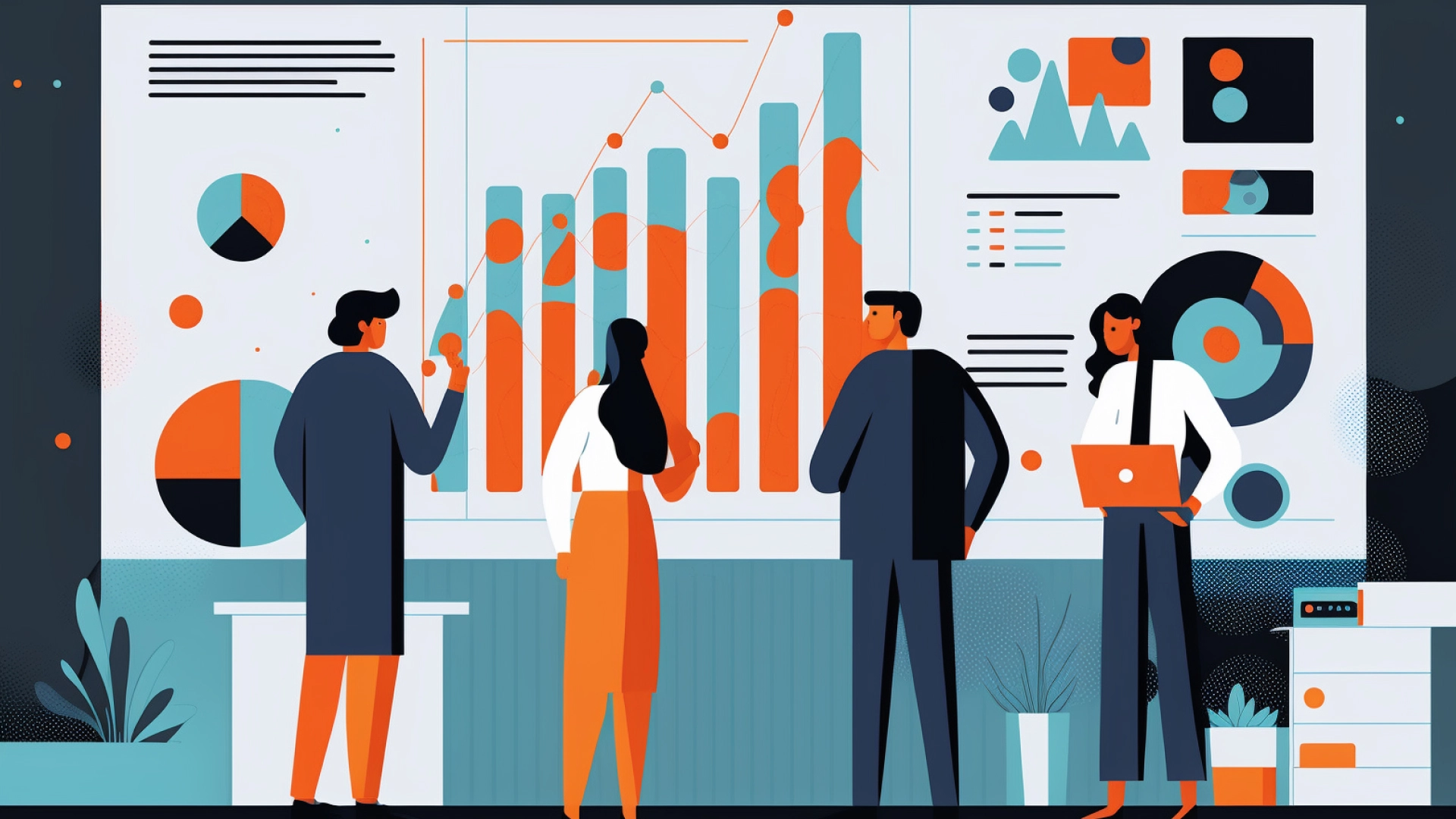
Thus, through analytics, healthcare can establish value-based care approach on every level of interactions with the end customer and improve both the quality of services and ROI. Some clinics are already doing it.
Cost management
Cost management analytical solutions cover different aspects of an issue. Some of them analyze patient data by the frequency of complaints and the severity of symptoms, hence hospitals can define top high-costs patients and prepare to treat them beforehand: buy necessary medication, prepare hospital beds and, of course, notify patients about their expected spendings.
Some approach the cost management from the pharma angle and process socioeconomic and medical data to provide patients with cost-effective treatment options. That makes healthcare friendlier and helps them manage the supply chain more efficiently, in accordance with their consumers’ needs and financial state.
Example. The platform amplicare.com syncs with a hospital’s pharmacy software and recommends medication accordingly to patients’ data. With it, hospitals make more accurate decisions in prescriptions and lower the DIR fees, avoiding generalised conclusions about meds.
Compliance verification
Healthcare is an industry that operates under a lot of restrictions and regulations, so it’s vital for medical institutions to operate in an ecosystem that is compliant to all of them.
Automated analytical solutions with a rule-based engine can check all documents that are added to a hospital’s database for compliance to HIPAA and FDA regulations and notify hospitals if something went wrong.
Example. coniferhealth.com created a program that connects to EHR and checks hospitals’ clinical documents in accordance with the new coding guidelines. It gets to the core of potential compliance issues and even provides them with training on a constantly changing ton of healthcare regulations.
Revenue cycle management (RCM)
Revenue cycle management in the value-based reality is more complicated than in the FFS. Within the latter, everything’s pretty strict and understandable: a service is followed with payment. The value-based approach requires more scrutiny: hospitals must optimize costs of services, detect and prevent frauds in payment data exchange processes — basically, to pay more attention to things that can harm their customers financially. Luckily, big data analytics simplifies that process and helps achieve in claims and payment, and detect mistakes quicker.
Example. CHS’s solution for hospitals’ RCM manages pre-registration, detects incorrect or missing claims before sending them to payers (which is really nice, because it reduces the number of denials), and manage denials themselves. Ergo, hospitals reduce errors in financial operations or detect them quicker. Fewer errors, more patient satisfaction.
Transforming through analytics is tough. How to deal with challenges?
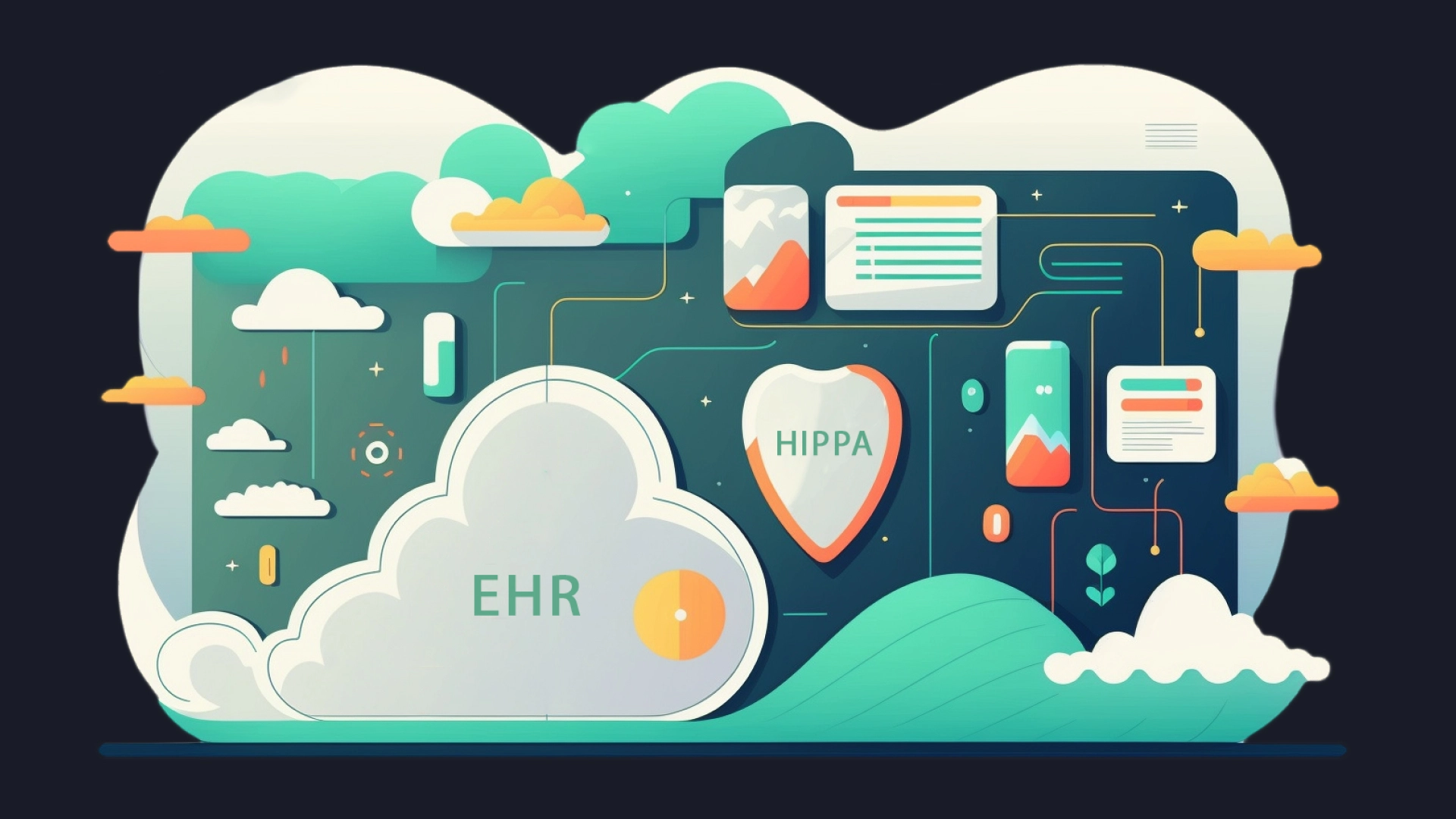
Healthcare providers, as well as third-party vendors who help them to unlock the potential of big data, surely, face a lot of challenges.
Let’s see how healthcare institutions and tech vendors, who plan to provide healthcare with engineering services can address them. (That's what we do when we build software for digital healthcare. You'll find these recommendations useful, too.)
If possible, choose solutions over tools
There is a lack of data scientists in every industry. In healthcare, that absence is severed by the fact that data science expertise usually has to be combined with some medical experience. Clinicians struggle with finding the right talent for the data-driven organization in the making. To beat that recession and gain maximum value out of analytics, we advise,
For medical organizations:
- Implement clinical analytics tools knowing that there’s a person with medical and engineering background that can analyze it properly and extract actionable insights out of it;
- If there’s no such person but you still want to use data insights to build value-based care, use analytics-as-a-service solutions which will present data analysis to you in a clear, understandable way with no coding needed.
For healthcare tech vendors:
Provide hospitals with solutions that can be natively embodied in EHRs and medical management systems — you will help them gain benefits out of data right away and save the efforts for medical analytics itself.
Think about regulations from the start
A tricky thing about the healthcare industry is that no matter how innovative your new tech solution is, if it isn’t compliant, it will bring you more pain than gain.
That’s why, for medical institutions and for third-party vendors who’re oriented on that market, our advice is to think about HIPAA, FDA, and HITECH regulations for data and device protection from the start, on the stage of concept of the solution (or before making a deal for hospitals).Also, implement a compliant data-protection strategy.
Data breaches are the number one challenge for data-driven healthcare organizations. 76% of security issues are due to hacking. Data strategy can be executed through the rule-based engine that “knows” all formats of patient data, emails, docs, scans, etc., and “protects” them—quickly identifies and reports inconsistencies, yet allows healthcare organizations to share data and establish a culture of knowledge.
Yeah, about that.
Endorse interoperability
Let’s say, Jane Doe, catches a cold during her Christmas holidays in New York but lives in Colorado. New York hospitals will have no knowledge about her previous interactions with healthcare. Maybe they’ll know her vaccination history. But that’s it.
To obtain a comprehensive image of patients’ data (a single provider’s data is usually not enough), hospitals work exhaustingly hard. Why? Because healthcare is hella huge and systems healthcare providers use are disconnected.
However, this will not be a case for long. In order to truly shift to value-based care, healthcare players (not only hospitals — pharma companies, insurers, and life sciences as well) have to create a pool of patient and research data. Better and easier access to data — more precise, data-driven decisions.
Hence, if there’s a possibility to connect data storages to other organizations, or move them to the cloud, or join some databank, agree to it. Before that, though, don’t forget to inform your patient on this decision, and check, if such connectivity is compliant.
Build comfortable user experience for patients and for doctors
User experience is important for healthcare, so it’s vital to convey the value of your services through mindful, human-centric interfaces — no matter who your end users are, patients or clinical workers. Implement the principles of universal design, build for edges, not for non-existent “average.”
Ergo, transmit the principles of precision medicine in building interfaces as well.
In fact, convey it through everything you’re doing.
It is a brilliant, smart, and rational approach that makes everything better.
Tell us about your project
Fill out the form or contact us
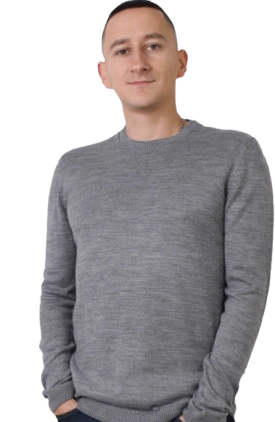
Tell us about your project
Thank you
Your submission is received and we will contact you soon
Follow us