
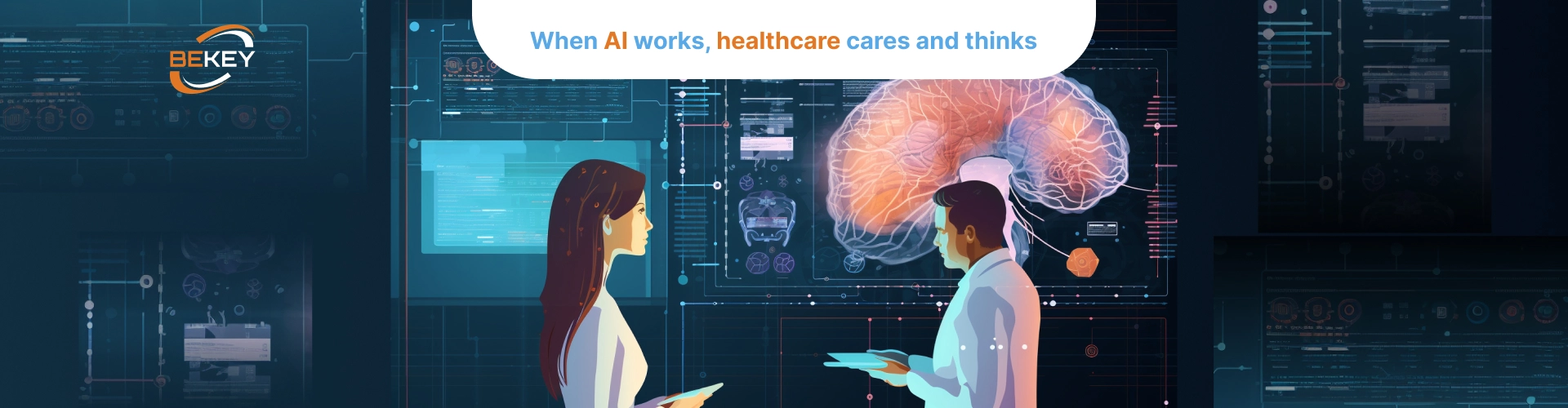
When AI works, healthcare cares and thinks
If earlier common thoughts about artificial intelligence in healthcare stand somewhere between fear and uncertainty, some doctors have already believed that machines will change the way care services are provided. Earlier, we’ve written about how big data algorithms help healthcare shift to value-based care. Now, let’s talk about recent AI developments — and how they’ll change the way care is delivered.
No-routine algorithms
We can talk a long time about how depthless, by thoughts of both clinicians and their patients, patients visits have become. Digitization ended up worsening communication in that interaction, instead of putting it in the center of healthcare. Artificial intelligence, along with automation software, is supposed to be fixing that: and it is. Natural language processing algorithms, combined with voice recognition technology should, sometime in the near future, free clinicians from keyboards and reduce screen time, spent in Electronic health records systems (EHRs).
According to Eric Topol, visit notes, recorded in that way, “transcend the typical quality of notes that appear in EHRs.” Evidence for this is already here: Nebraska Medicine, a healthcare provider in Omaha, decided to integrate Epic (one of the most popular EHRs in the USA) and Dragon Medical One, a voice recognition platform from Nuance Communication. Results are stunning: there was an improvement in documentation quality and reduction in transcription costs; most caregivers were enabled to do their jobs better and half of them fight 30 minutes of their workday back - https://www.healthcareitnews.c...
If digitization and widespread usage of EHRs started with “go paperless” slogan, software that will leverage AI for caregivers will probably be reimagined under “back to eye contact” motto.
AI as a fight buddy in diagnosis and treatment
Some advancements in how machine learning algorithms help increase efficiency and accuracy of diagnosis and treatment, as it seems to us, appear almost every day. Surely, AI is not perceived as a trusted partner by the majority, but it definitely already helps healthcare organizations who were willing to take risks and researchers.
A bit from recent news: scientists and IBM combined machine and deep learning and trained the algorithm on mammograms and health records to detect signs of breast cancer better. The algorithm learned to predict the results of the biopsy and to distinguish between normal and abnormal screenings. Then, the results were compared to risk factors for breast cancer: for instance, the program was able to link persons’ medical images to results of her blood and thyroid function tests. AI also learned to detect almost half of false-negative findings on the images. Its sensitivity reaches 77.3%.
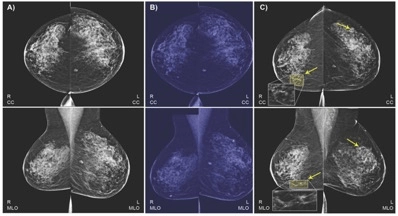
Same screening quality improvements, provided through the use of AI, are shown in the detection of the early signs of colorectal cancer — precancerous polyps, an adenoma. “For each 1% increase in the adenoma detection rate (ADR), the risk of developing colorectal cancer within five years of a colonoscopy is reduced by 3-6%,” says UCI Health, reporting about the research.
AI is already fighting with clinicians for accuracy and precision — last August, Google’s Deepmind researchers collaborated with Moorfields Eye Hospital and trained its software on scans of patients with retinal diseases. The algorithm was able to detect more than 50 cases of disease and recommend treatment for it.
Another thrilling news about the application of AI in healthcare is in pharma. Recently, Australian researchers released the first flu vaccine, developed by a machine. Jokes aside: Flinders University scientist “fed” to machine learning algorithm data on compounds that awoke the immune system and dataset with compounds that are ignored by it. Another program processed all these and selected the best-fitting candidates, and it’s occurred that program obtained information not only about existing drugs, but also new ones, missed by the human eye — and here we are. The clinical studies for it are yet to be conducted.
So, AI definitely helps healthcare and will help greater deal in the future: both in terms of prevention and accuracy improvements — and in terms of finding patterns and new treatments, outcomes, and solutions to discover for healthcare providers.
AI for self-care and self-diagnosis
When the routine is eliminated or reduced and caregivers can make smarter, more comprehensive decisions, the quality of care increases — and patients get personalized, accurate treatment. The advancements of AI and its perspectives want to reach patients where they are now and relocate the initial point of interaction with a healthcare system from the visiting room or ambulance to patients’ home.
Combined with wearable devices (which soon have to become more affordable and accessible), AI algorithms can turn real-life data into reports about people’s conditions and changes in it — no invasions required. Patients with chronic diseases will get updates on their ECG and glucose level, and these data will be sent to their caregivers.
Some providers have already started transforming the way they work with patients. NHS Glasgow, for instance, implemented a project aimed at fighting Chronic Obstructive Pulmonary Disease (COPD), one of the most common causes of emergencies over there. Right now, they are using a wearable ventilation mask that collects real-time data about patients’ breathing. Clinicians who are getting that data through cloud may adjust the settings of the mask accordingly to monitoring — and communicate with patients through an app. Right now, trials have shown a significant reduction in hospital admissions for COPD reasons — and if NHSGGC’s solutions will work on scale, it would mean savings for hospitals and better care for their patients.
Another solution that caught our attention is a mHealth app that can detect ear fluids and, therefore, ear infection through smartphone’s microphone, speakers, and a regular piece of paper in a funnel form. The trick is in the soundwaves: if patients have an ear infection — fluids in their ears, — sound doesn't resonate fully and reflects sound back. If they don’t, a “music”, detected by microphone sounds differently. AI here comes at recognizing different sounds: it can tell exactly where a fluid is located and how much of it is in an ear.
Another frontier for healthcare and AI symbiosis advocates is access to open data. Quality of AI forecasting largely depends on how comprehensive the obtained data picture is — and if patients own their data, not only from hospitals and clinics, but also from wearables and, maybe in the future, their home, — the awareness of what’s going on with their bodies and, therefore, their health management will improve drastically.
Greatest AI accomplishment-to-be in the healthcare
It is hard not to get tremendously excited about AI opportunities when almost every day, the science community or healthcare startups release reports on how some algorithm made “X” simpler and easier — and some of them are described above.
The thing is, though, that accuracy and precision and even out-of-box solutions that are being delivered through the artificial intelligence can’t be compared to the human touch that was drained out of healthcare at some time between sending a fax and filling up patient information in EHRs. And that is what AI will do best. While all the novelty and science and even hype — look at how many people are talking about healthcare — are good, the improvement of patient—caregiver relationships is better.
That being said, it’s absolutely clear that AI has no chance to replace humans in healthcare settings — it’s unlikely that doctors’ responsibilities will be somehow limited to emergencies.
There’s a term "deep medicine", coined by Eric Topol in his book of the same name. It’s defined as a combination of deep phenotyping (as Topol explains, “all [data] layers that make us tick”) and deep learning that is used to provide empathetic, personalized care. Being one of the first pioneers of AI applications in healthcare, he believes deep medicine has the potential to improve patients’ health outcomes, improve their well-being and give doctors time and the most enjoyable parts of their jobs back.
We think that concept sounds amazing and we want more healthcare businesses to step into it.
AI’s “buts” and what health tech can do about them
There will be no value-based care without tight collaboration between technology vendors and organizations that want to adopt a technology.
The most thriving challenge standing between healthcare and life sciences and data and AI businesses and startups is that... former don’t recognize the need in it. The second one is that healthcare has only 2.4 / 5 points of “data readiness” (for being presented as a data set for machine learning or deep learning), and a lot of organizations think they have too few or too low-quality data.
Lack of talent is also persistent, and data scientists and engineers aren’t the only ones missing, — healthcare and life sciences organizations also feel the absence of people who are able to build use-cases for AI and connect them to business goals.
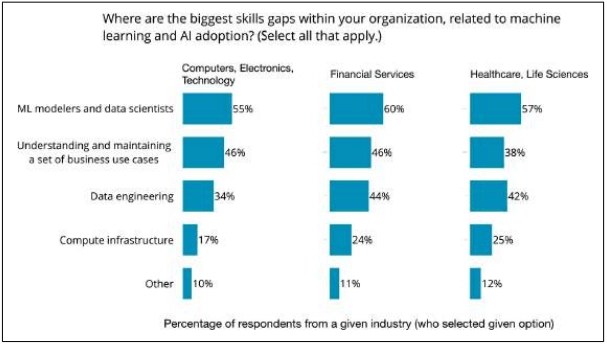
Among the major fears, uncertainty in machines’ predictions, fears of bias and ethical issues, safety, and transparency.
People who work as a healthcare tech providers must recognize these prepositions: healthcare itself isn’t the only point they are touching. Healthcare isn’t a place for tech innovation hurry — and, despite the fact the industry’s AI investments are huge, one step at the time is still the best strategy, both for pilot projects in large tech organizations and for startups.
Clinical trials, controlled and randomized, is the only evidence you need to reduce the fear of uncertainty — but if you miss it, you’ll never be able to regain trust.
Medical data are never perfect and never suitable enough, and the algorithms should be built with that thought in mind.
Business cases — connection of AI adoption on primary care level to revenue cycle management — aren’t obvious if you don’t have the numbers, and that what healthcare decision-makers are thinking about.
While healthcare community thinks about what to implement to make patients’ lives easier and their health better, healthcare information technology community should, in our opinion, think about the life cycle of their AI products, and their adoption, and their future role in people’s work and lives.
Tell us about your project
Fill out the form or contact us
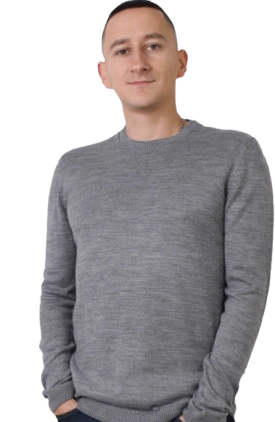
Tell us about your project
Thank you
Your submission is received and we will contact you soon
Follow us